MICROSOFT SOURCE
Tiny but mighty: The Phi-3 small language models with big potential (opens in new tab)
April 23, 2024
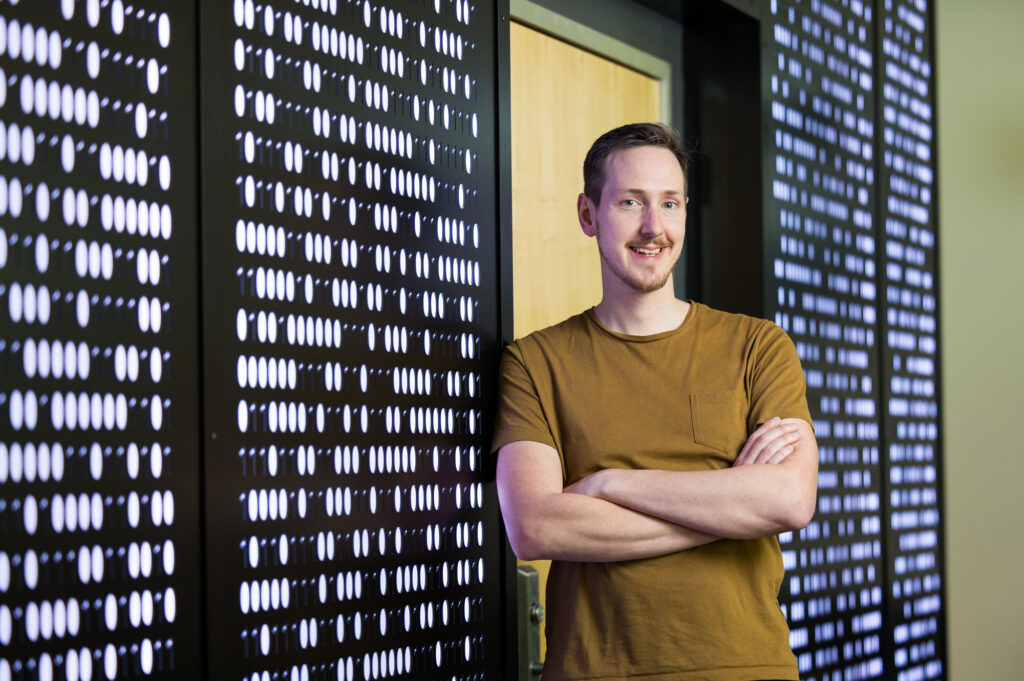
Microsoft Research Blog
Ideas: Exploring AI frontiers with Rafah Hosn
April 25, 2024 | Rafah Hosn, Gretchen Huizinga
Microsoft Research Blog
SAMMO: A general-purpose framework for prompt optimization
April 18, 2024 | Tobias Schnabel, Jennifer Neville