MICROSOFT SOURCE
Tiny but mighty: The Phi-3 small language models with big potential (opens in new tab)
April 23, 2024
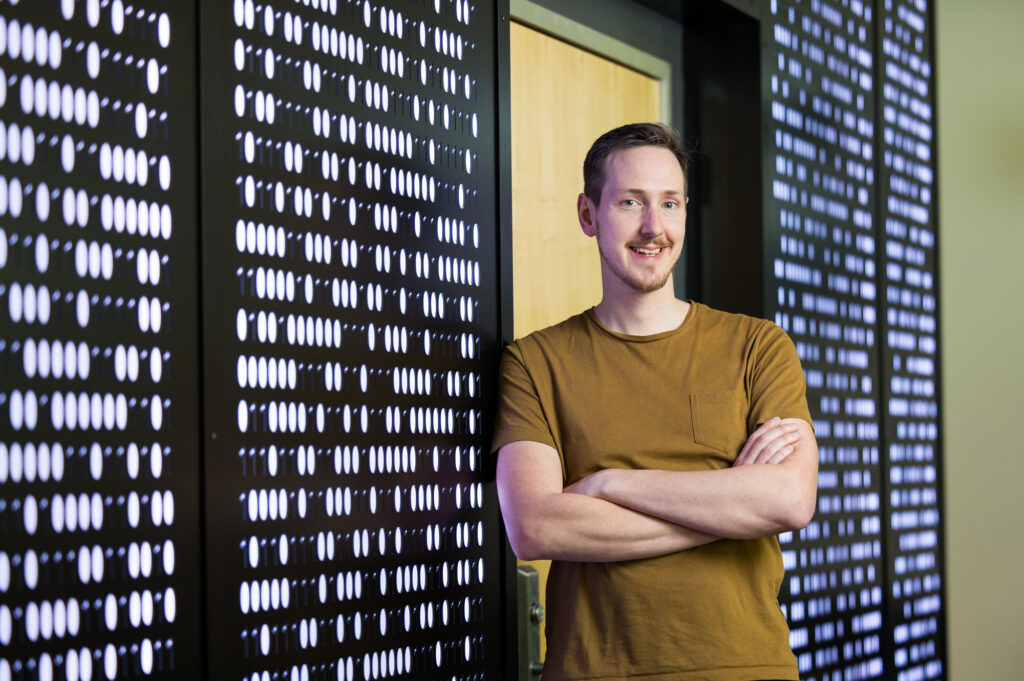
Microsoft Research Blog
Microsoft at ASPLOS 2024: Advancing hardware and software for high-scale, secure, and efficient modern applications
April 29, 2024 | Rodrigo Fonseca, Madan Musuvathi
Microsoft Research Blog
SIGMA: An open-source mixed-reality system for research on physical task assistance
April 29, 2024 | Dan Bohus, Sean Andrist